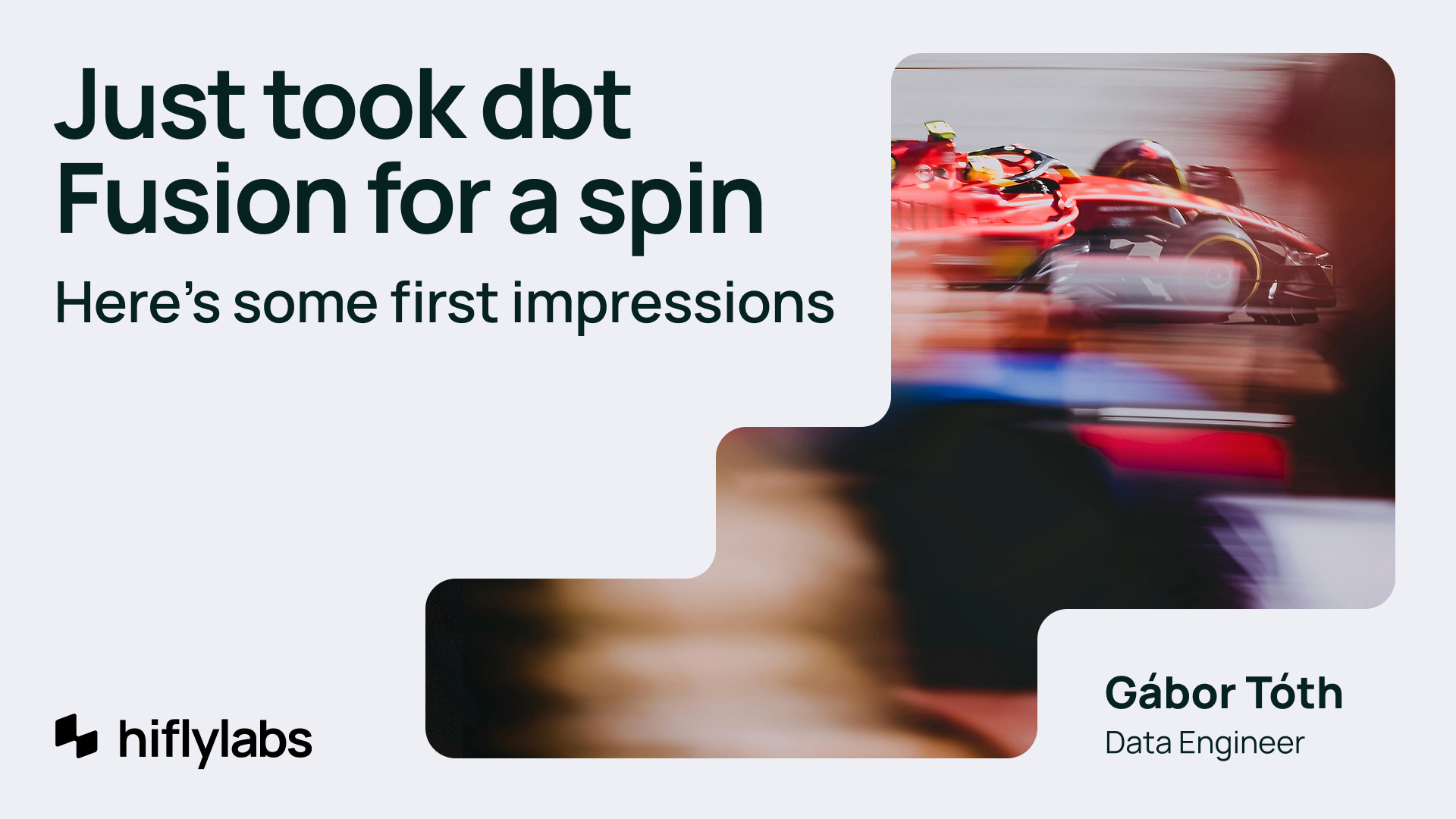
dbt Fusion: A First Look and Hands-On Review
dbt Labs recently announced dbt Fusion, a complete overhaul of the dbt Core engine built in Rust. It promises to significantly improve the developer experience. In this article, we test its core features and share our hands-on experience with the public beta, exploring what works, what doesn't, and what potential it holds for the future of dbt development.